Using AI To Your Advantage in Banking & Finance
In order to stay competitive, banks and fintech firms are under strain to incorporate new technology.
Financial services companies may use AI to automate procedures, boost productivity, save costs, and enhance customer service.
The banking sector already employs AI for several functions, including identifying fraudulent transactions, NLP, visual image identification, and predictive modeling.
Financial institutions may outperform their competition by investing in machine learning technologies.
Customers that are tech aware and often interact with cutting-edge technologies want banks to provide smooth experiences.
For services like phone banking, e-banking, and real-time money transfers, banks have expanded their industrial landscape to include retail, IT, and telecom in order to satisfy these demands.
While these developments have made it possible for clients to access the majority of banking services at any time, anywhere, they have also come at a cost to the banking industry.
What Impact Does AI Have on the Banking Sector?
In the recent decades, the banking industry has seen a stunning digital change.
That may come as a surprise given that financial organizations are typically associated with rigorous methods and inflexible structures, mostly because of security-related concerns.
Globally speaking, nevertheless, they have quickly achieved a notable progress.
Who would have imagined that twenty years after contactless payments were introduced, we would be able to buy goods without carrying a card or even a mobile phone?
Or quickly complete the overseas transfers without the need for a central hub to operate as a go-between?
The advent of artificial intelligence has led to many changes in the banking industry, and as time goes on, more and more procedures in financial institutions and applications will be handled by AI tools.
The effects of AI are readily apparent in many aspects of the market, from forecasting to customer experience to decision-making procedures.
It's interesting to note that although some Western nations shunned these prospects, others, especially those that were once part of the Soviet Union, like Poland or Estonia, have emerged as the forerunners in financial innovation.
Their financial institutions have recently used cutting-edge technologies and tactics in their mobile apps and customer support, establishing new European or even international standards. This is a route to offer the best digital transformation solutions.
There is currently no turning back since both businesses and their customers have gotten accustomed to the ease that AI products offer.
Most likely, the banking industry will keep embracing AI's potential, especially in the areas of fraud detection and decision-making, and fusing it with other cutting-edge technologies like Blockchain, which are currently gathering traction in the financial world.
Will the market adopt the newest AI-based technologies, such as contentious face recognition for payments?
Time will tell, but there is little question that artificial intelligence will continue to transform the market and encourage a focus on the needs of the client.
If you also have an idea you wish to implement using AI technology, you may get in touch with TransformHub, offering top digital transformation services in Singapore.
AI Applications in Banking and Financial Services
AI applications in banking might be internal or external, much as in other sectors.
On the one hand, artificial intelligence technologies improve the organization's security, a key component of financial services, and expedite internal procedures (including decision-making).
On the other hand, the quality, speed, and accuracy of financial services are positively impacted by AI technology, which enhances the client experience.
1. Services for personalized bankingIn many sectors, personalization is a potent weapon, but it is most effective where the success of a market depends heavily on the satisfaction of the client.
It seems to make sense that banks have begun to make use of it to make their services more competitive.
In the end, banking products are rather uniform; it is difficult to differentiate an offer by its price or by its terms.
Personalization emerges as the institution's hidden weapon in such a setting, enabling adaptation of the message and offer to the preferences of a specific consumer and thus raising conversion rates.
The machine learning algorithms may collect client data and process it for customized purposes, enhancing its accuracy over time. This is made possible by their recursive learning capabilities.
Utilizing historical data, prescriptive customization creates workflows that are optimized.
The development of individualized suggestions and the customization of the virtual assistant's assistance based on natural language processing models, on the other hand, need real-time personalization, which uses both past and current data.
Customers enjoy feeling special and heard, and the AI-powered customized virtual assistants deliver that feeling.
As a result, it's the ideal method for inspiring consumers to take action while also lowering their effort.
AI, thus, plays a major role in implementing digital transformation solutions.
2. Detecting fraudYou may have recently learned a lot about Blockchain in terms of preventing fraud.
And in fact, fraud is considerably less likely to happen because of the openness and inviolability of the blocks inside the blockchain network.
However, machine learning-based methods must be used by financial organizations in order to properly identify it.
Naturally, combining the two will yield the best results!
In order to identify fraud, banks can use algorithms that are unsupervised in their systems.
They are trained on past data and can spot odd trends, which speeds up the review process and replaces certain human tasks.
The algorithm can supplement manual verification by preselecting any discrepancies that will be checked by a real-life expert in the next phases. Manual verification is still essential.
Leading financial institutions can lower the cost of activities linked to fraud detection and boost their efficacy, lowering the likelihood of human error.
Various fraud tendencies can be recognized by machine learning algorithms in financial transactions:
- Group of Abnormalities
- Conditional Irregularities
- Point Deviations
Due to the wide range of potential anomalies and noise, which frequently results in many false positives, developing an efficient machine learning (ML) model for fraud detection (typically based on logistic regression, a technique that enables effective grouping of the suspicious actions, but occasionally also takes time.
3. Assessment of loan risk and credit scoringThe banks may automate their credit scoring and loan risk assessment processes using supervised learning (mostly logistic regression models), which makes the processing of loan applications quicker and more efficient.
To determine loan risks and make credit judgments, statistical models examine the demographic and behavioral data.
Other risk-defining variables, such as spending patterns, can be considered by the algorithm in addition to age, address, monthly income, house status, and length of work.
A credit score will be sent to the potential lender, which will be used to inform their choice.
Banking organizations can use previous data on loan defaults and successful repayments to educate their algorithms.
They gain the ability to recognize trends that point to potential default, enabling more precise risk assessment.
The program will raise a red alert if the account history demonstrates trends that indicate danger (such as a lack of regular saves, large spending, or erratic incoming transfers).
In a similar vein, the consumer will have a better probability of being approved for a loan if the patterns suggest dependability.
Predictive algorithms can assess the interest rate risk and prepayment risk in addition to default risk, which are crucial elements in loan underwriting.
4. Forecasting to increase bank incomesBanks employ predictive modeling to spot possible risk in the loan underwriting and fraud detection processes, but the possibilities don't stop there.
Many institutions seek it out in an effort to increase cross-selling effectiveness and boost sales.
For a specific client, the predictive models may learn from historical customer behavior data to develop more pertinent cross-selling offers.
The suggestions may become even more precise, driving more sales, with a comprehensive segmentation of the dataset and market basket research.
5. Using face recognition to facilitate frictionless paymentFinally, we get to talk about one of the most contentious uses of AI in banking: face recognition for financial transactions.
Such payments are processed by customers’ systems, which link them to a customer's DNA to keep track of their preferences and deliver more precise suggestions each time.
This computer vision-based technique is rather straightforward: after scanning your face, the payment terminal sends its template to the interpreter, which checks it with the certified template from your bank.
6. Enhanced client servicesTo keep one step ahead and deliver the most precise answers, the VA analyzes the account details, demographic, and behavioral data after validating the client's identity using cutting-edge methods like voice biometrics.
The buyer may avoid getting into the specifics in this way, saving valuable time. The quick data check that VA does allows it to identify the inquiry's potential origin.
The customized suggestions made based on client data may nevertheless increase conversion rates even if it's a miss.
The customized virtual assistant may resolve a variety of problems, including many more!
By establishing a connection with the CRM, they may issue and handle papers as well as schedule appointments with actual consultants.
7. RPA and NLP-based back-office process automationWhile the aforementioned uses are impressive, it should not be forgotten that banking institutions also receive help from artificial intelligence.
Banks can employ robots to automate routine, time-consuming, and prone to human mistake tasks like processing payments and invoices using robotic process automation (RPA).
Consequently, company costs drop, productivity soars without adding more employees, the rate of invoice mistakes declines, and process execution speeds up dramatically.
Banks can instantly generate a large number of invoices and arrange their delivery, thanks to RPA.
It might also be used to streamline collections, secure invoicing, and review payments.
The bots can extract data and learn from documents using natural language processing (NLP), which makes it easier to analyze applications and make decisions.
How Can AI Give You a Competitive Advantage?
The potential benefits of AI should be embraced as soon as possible, but only after a careful examination of the organization's structures and procedures.
By doing so, you'll be able to spot possible improvement areas and integrate AI solutions that are truly compatible with your company strategy.
Let’s Connect!
We at TransformHub, counted among the best digital transformation services companies, are here to take complete accountability to deliver precisely tailored solutions based entirely on your business requirements.
Get in touch with us today to turn your vision into reality!
Share this
You May Also Like
These Related Stories
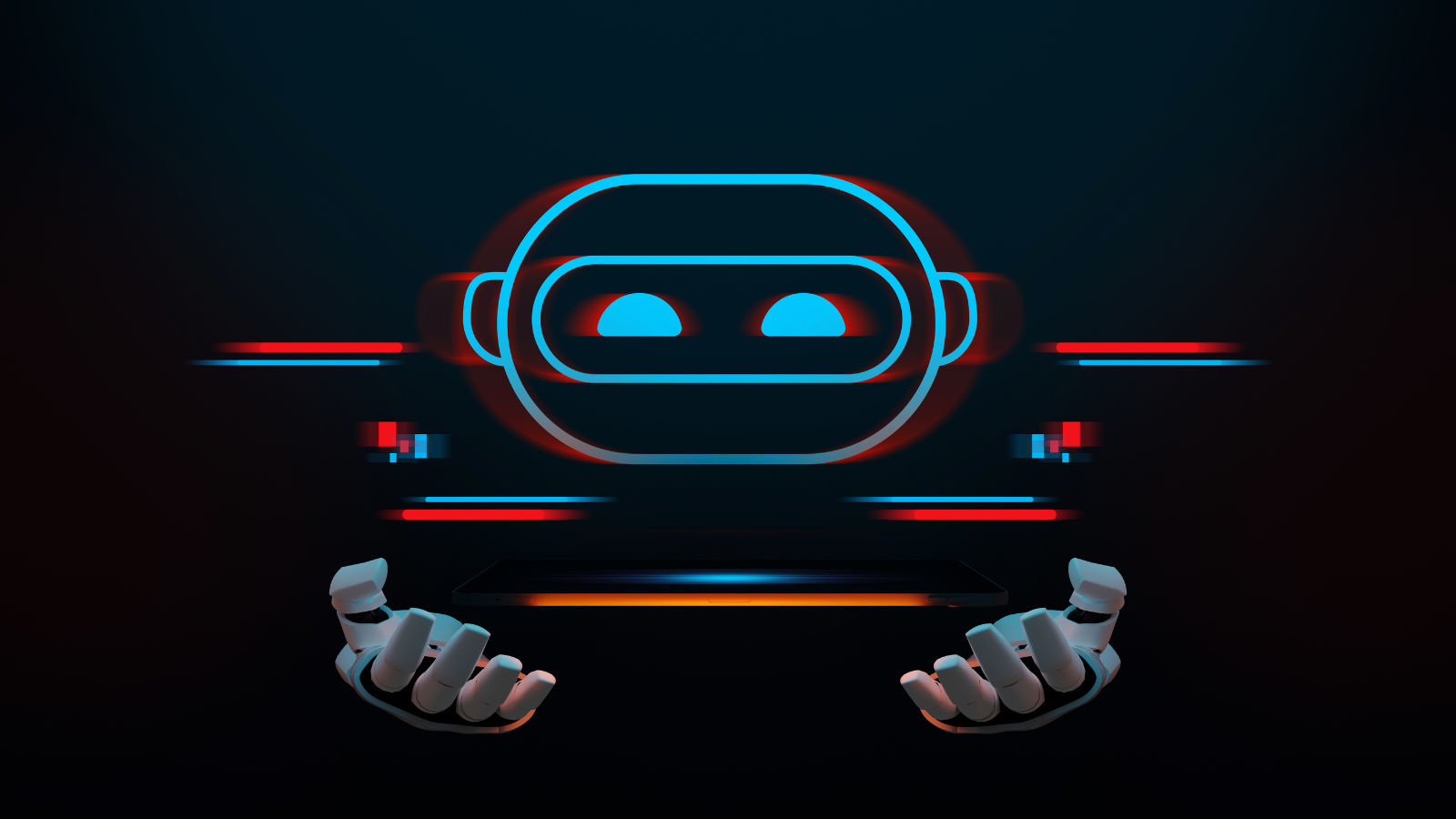
Generative AI and What it Means for Businesses
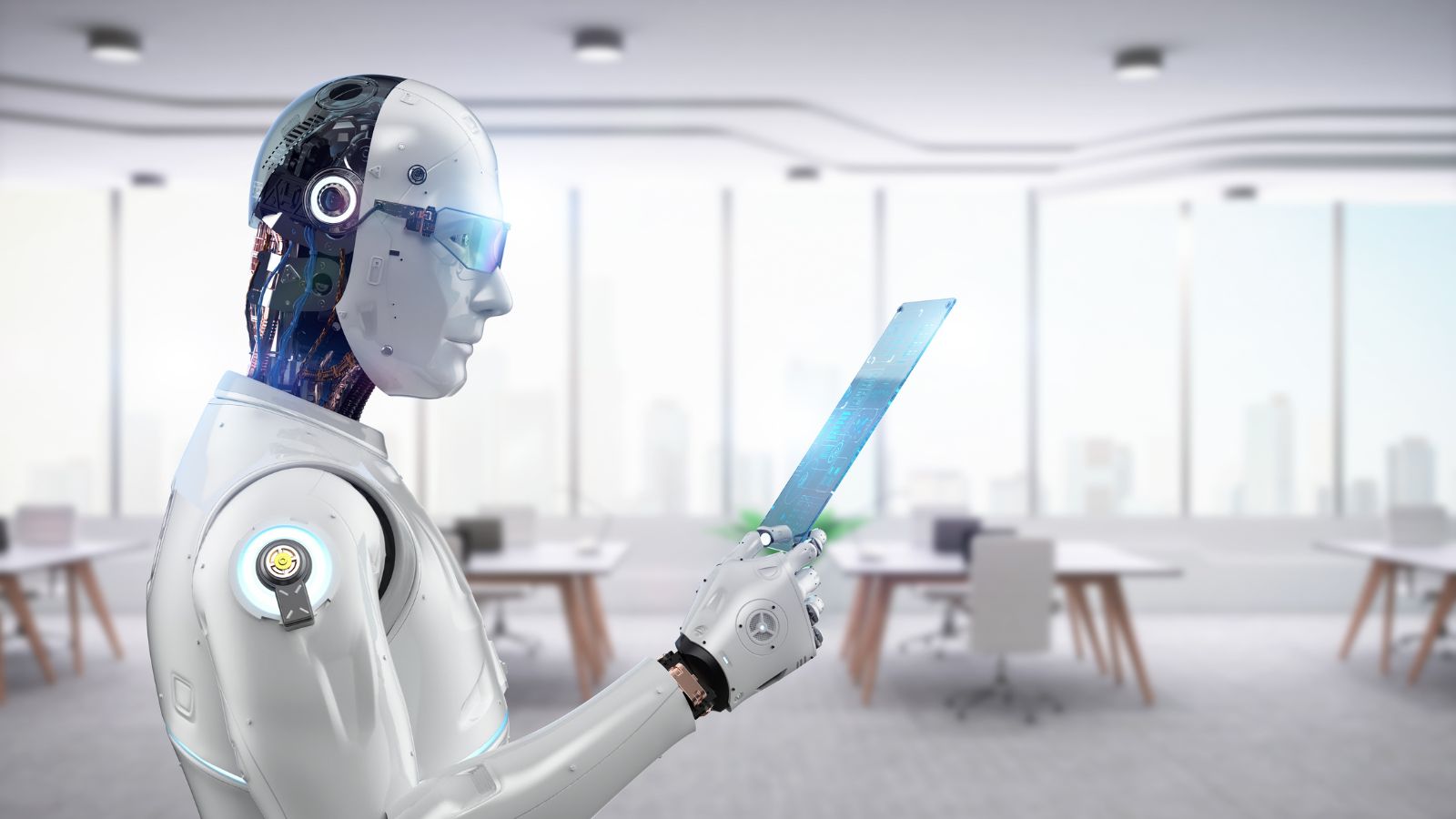
AI for Digital Transformation
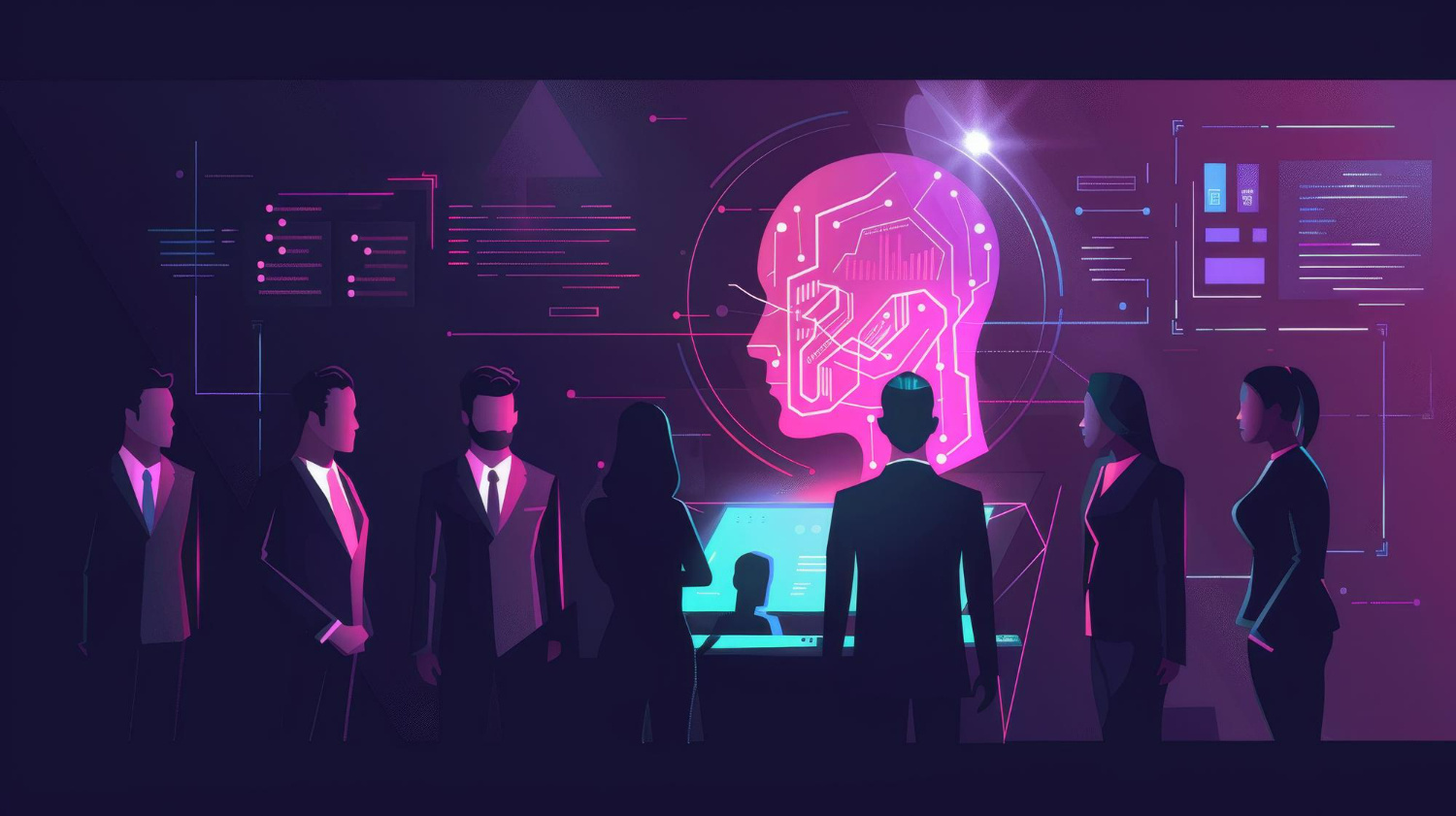