How AI and ML Are Changing the Lending Ecosystem
We now live in a digitalized world where technological improvements have helped people & businesses flourish & remain ahead of the competition. During the past several years, a plethora of mobile loan applications have exploded. Due to the officials' encouragement of banking digitization as a result, fintech companies sprang into action to close any gaps, especially in the area of digital loans.
Artificial intelligence (AI) and machine learning (ML), two disruptive technologies, are proliferating in practically every industry. The financial sector isn't far behind and has a lot of data. Using these technologies, they created goods that matched the changing requirements of their customers. By enabling more precise and quick decision-making through analysis of consumer trends and patterns, machine learning has caused a stir in the lending industry.
As a result, machine learning (ML) is a branch of artificial intelligence (AI) that employs sophisticated algorithms and statistics to carry out certain tasks digitally and in real time by examining enormous data sets.
Lending companies may quickly and concurrently detect, classify, and make precise judgments based on many data sources with the aid of AI and ML as they opt for digital transformation solutions.
The Role of AI and ML in Lending
Unlike ML, which is more of a subset of AI, AI is a whole branch. It's commonly referred to as a "device" or "process," depending on the context. Together, AI and ML are viewed as a system or form that can mimic human intellect.
They not only handle data quickly, but they also do it more accurately and impartially. They become an invaluable resource in the financial services sector, particularly in lending, which receives millions of applications but frequently cannot handle them all owing to prejudice on the part of people, a lack of resources, and mistakes.
AI may be divided into supervised and unsupervised categories.
Supervised AI: Large banks with access to a lot of data and their own "lending criteria" are where you'll often find supervised. Based on these preset criteria, supervised AIs are entrusted with sorting through the enormous quantity of data and applications.
Unsupervised AI: It is better suited to smaller banks and lending organizations that do not have rigid regulations in place and are okay with a less rigid sorting structure. They have the advantage of producing more individually tailored loans and programs for their clients as a result.
A data scientist must input a lot of data (Big Data) to set up an unsupervised AI and let the AI function. The enormous amount of data is then read by AI, which uses several factors to uncover structures and patterns in the otherwise chaotic arrangement. Machine learning assists AI in doing this task.
Based on the patterns that AI recognizes, it performs a range of "if-then" activities using algorithms and different statistical models. Many companies use a bigger dataset as its input (often referred to as training data) through Machine Learning, processes it through its algorithms, and then outputs conclusions or predictions.
When it pertains to lending, the ML method will let the lender make a choice on whether to grant an applicant a loan of a specific amount, length of time, and interest rate based on the applicant's data and that of individuals who fit their profile.
As a result, lenders have a far better grasp of the types of loans to offer to ensure client pleasure and maximize profits.
Bias is eliminated from the entire loan process through ML
At first, loan origination was a labor-intensive, entirely manual operation. The reliance on human involvement affected outcomes as well as pace. Human bias would frequently seep into the underwriting process, causing application rejections or higher/lower interest rates to be applied to some loans, which would result in consumer displeasure or loss for the lender.
ML has eliminated this prejudice by churning the applications through its algorithms to hunt for patterns and give insights and conclusions based on the same; no emotions are involved. This lowers mistake rates and produces loans that are more profitable for lenders.
How Have AI and ML Changed Lending Substantially?
More individuals are now using banking services as a result of increasing financial literacy and awareness, but more significantly as a result of access to banks and the internet. In addition, they are more willing to apply for loans at banks since they are more familiar with how institutional loans operate than indigenous lenders who would demand higher interest rates and exhibit little flexibility.
Lenders are now forced to offer loans to borrowers with little to no credit history in order to remain competitive in the market, since more applications are coming from new entrants to the banking system.
The banking and lending sectors have advanced significantly since 2015, when Javelin Strategy performed research that revealed 15% of cardholders had at least one transaction mistakenly refused, costing banking corporations $118 Bn in lost card sales.
This issue was solved in large part because of AI and ML. By leveraging an applicant's digital footprint, search queries, and social media hygiene to determine their creditworthiness in the absence of a FICO score, it is possible to reduce mistakes in loan processing and approve transactions.
Lending institutions have benefited from using ML to maximize each application they get. Another victory for the lending sector comes from automating the challenging loan origination process and simplifying it to deliver outcomes in a couple of days.
Customers are happy and lenders' revenues and profit margins are stronger when they process loans more quickly. In the era of AI and ML in lending, customer satisfaction is no longer an urban legend but rather a truth.
Benefits of AI & ML in the Lending Industry
1. Quicker KYCConventional KYC procedures need manual labor and a lot of time; AI can simplify this process. Customers' data is studied to identify trends in their behavior, and loans may be tailored to each individual's needs, giving lenders access to a captive market. In terms of customer service, chatbots powered by AI guide numerous consumers at once to the required items while providing fast assistance.
2. Credit score generationThe worth of a loan is based on the borrower's or lender's business' creditworthiness. In order to assess a person's creditworthiness, algorithms supported by ML technologies sift through a tremendous amount of data from social networks, mobile devices, payment systems, and web activity.
A credit score is generated by looking at a potential borrower's whole digital footprint and is used by lenders to determine the loan value. The quick decision-making reduces the turnaround time for processing loans substantially.
3. Fraud detection and risk managementLoan stacking is a frequent practice in the lending industry where borrowers take out numerous loans from various lenders. Lending applications require AI and ML capabilities to profile client behavior and detect questionable trends that might result in fraud utilizing massive volumes of user data and transactions.
Lending companies may make educated judgments thanks to the actionable knowledge that ML technology's insights give. ML-enabled algorithms can identify clients who are likely to default on their loans and work with lenders to change the conditions of loans.
4. Reduced costsFintech and digital lending companies have business models that are supported by technology and need little to no human interaction, which lowers operating expenses.
Online submission of the required paperwork eliminates the need for physical submission and allows for virtual verification and evaluation, which makes the process more effective.
It is simple to check the applicant's whole credit history and capacity to make payments on time through their digital footprint. However, manually predicting and modifying a borrower's behavior takes a lot of effort and is prone to mistakes.
What’s Next?
Machine learning is now essential to many parts of the financial ecosystem, including asset management, risk assessment, investment advising, combating financial crime, document verification, and much more.
We believe that adopting artificial intelligence and machine learning in lending and international finance in general is the suitable pathway to achieve the same. As financial awareness and financial inclusion among consumers from various geographies and all walks of life increases, it is essential that lenders develop the successful technique to sequence & extend financial lending tools at scale & in a cost-effective manner.
When dealing with a variety of tasks, ML algorithms are continuously learning from vast amounts of data and bridging the gap by advancing the development of a fully automated financial system.
For the loan sector, AI and ML are no longer only a possibility to contemplate. The current state of the sector confirms this. The sooner lenders get on the AI and ML bandwagon, the better their prospects are to capture a lucrative market share and attract clients with markedly higher value.
With the support of TransformHub's experience in utilizing cloud technology, fintechs may quickly achieve organizational agility and scalability.
In order to obtain the acceleration your fintech firm requires and learn more about our services and offers, feel free to connect with us.
Share this
You May Also Like
These Related Stories
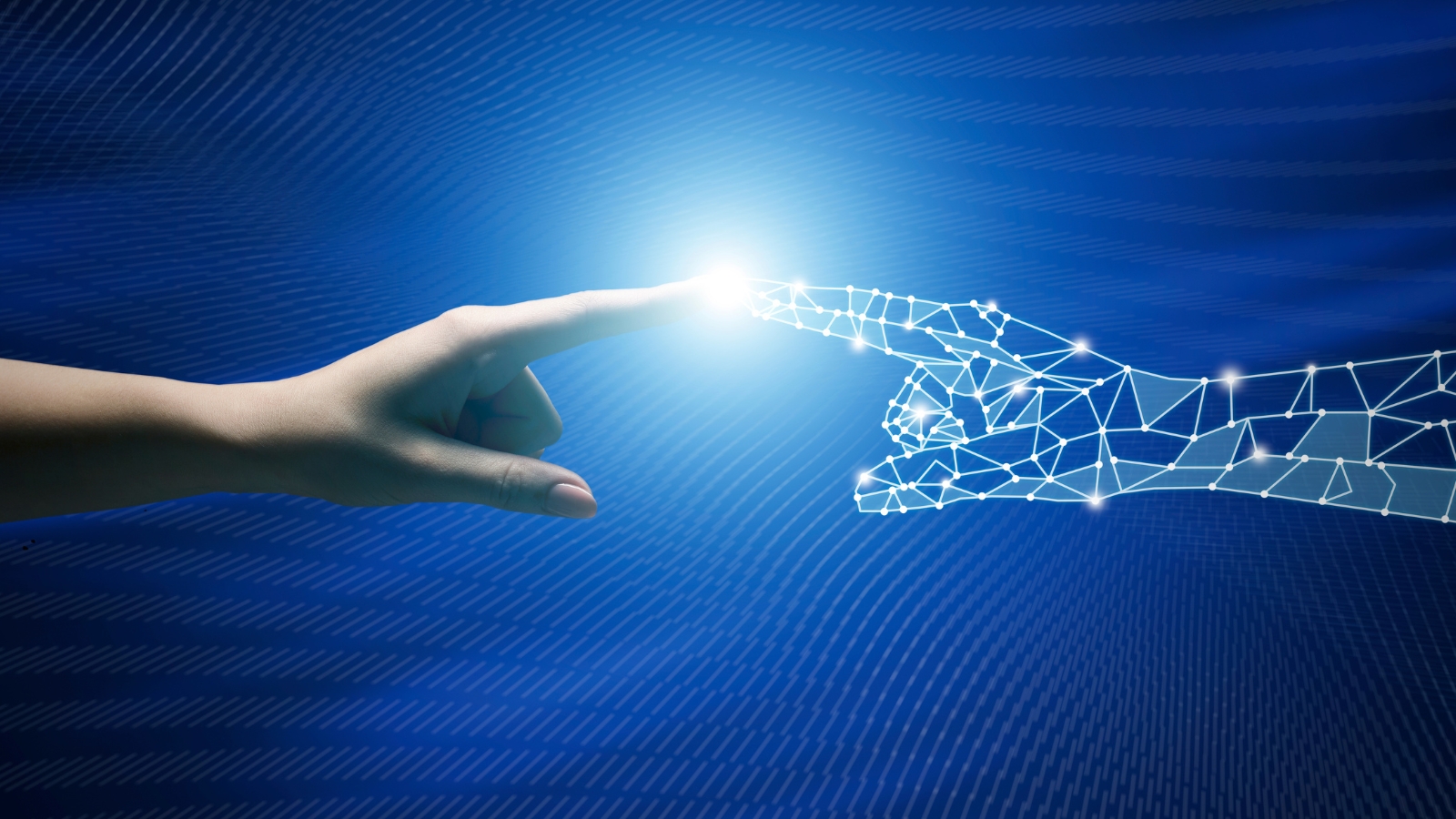
Where Business Intelligence Meets Artificial Intelligence
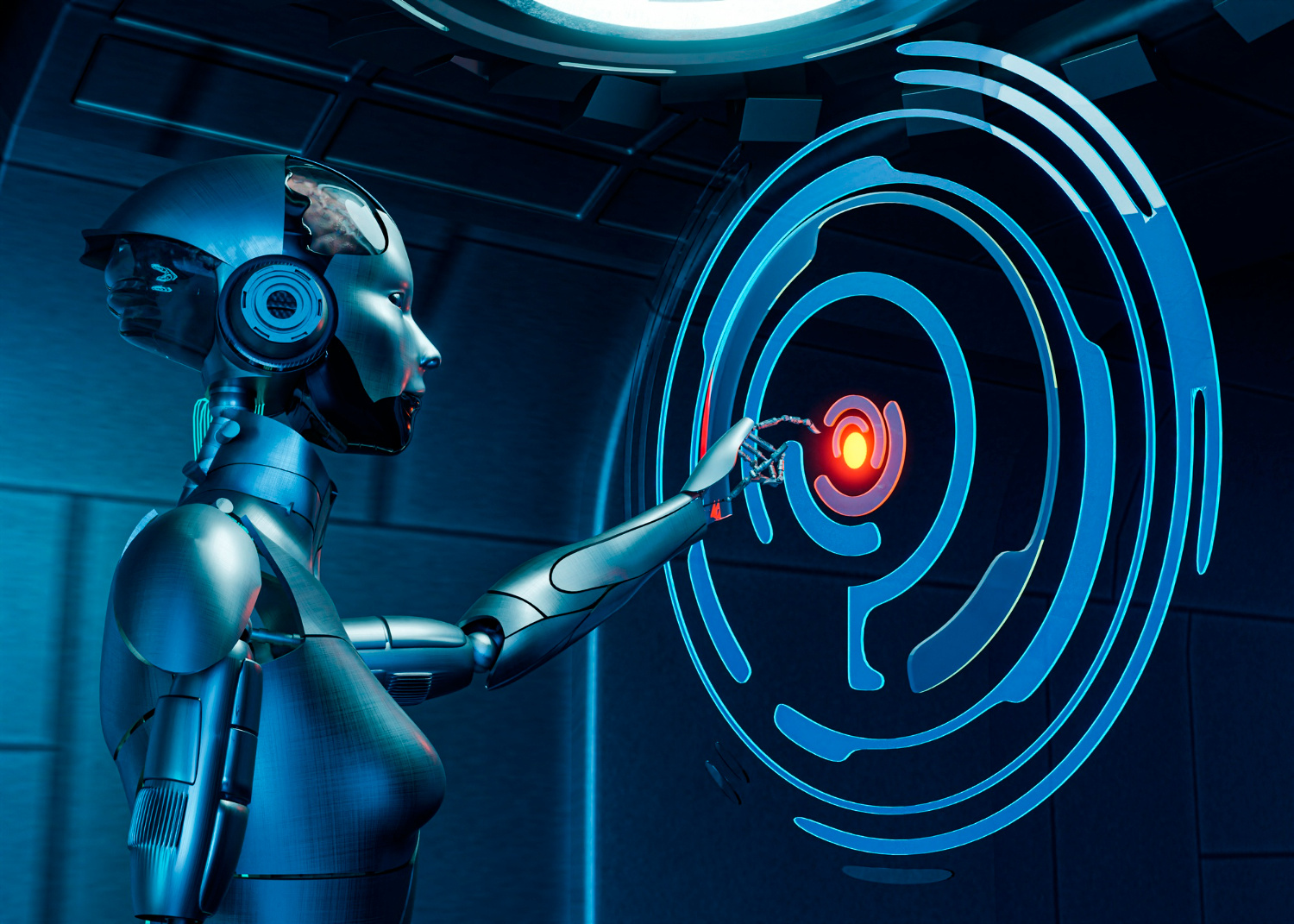
AI in Risk Management: Proactively Identifying Threats
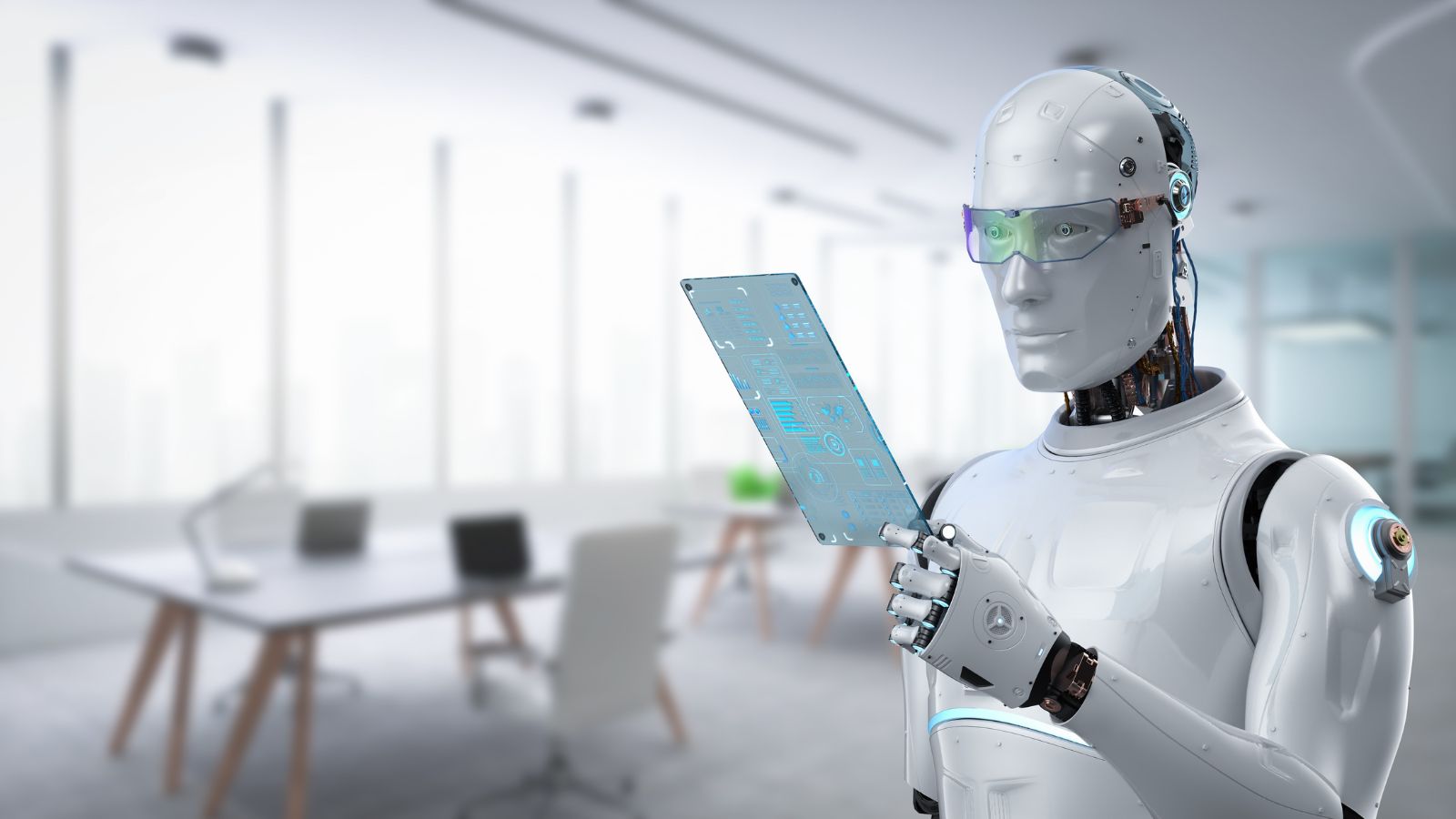