Generative AI: The What, The Why, and (Most Importantly) The How
In the rapidly evolving landscape of artificial intelligence, Generative AI has emerged as a revolutionary force, pushing the boundaries of what machines can achieve. In this comprehensive exploration, we will delve into the fundamental concepts of Generative AI, unravel the reasons behind its growing significance, and most importantly, demystify the intricate process of how it works.
The What: Understanding Generative AI
Generative AI, short for Generative Adversarial Networks (GANs), is a subset of artificial intelligence that focuses on enabling machines to generate content autonomously. Unlike traditional AI models that rely on pre-existing data and patterns, Generative AI has the unique ability to create new, original content based on its understanding of the input data.
At the heart of Generative AI lies the GAN framework, introduced by Ian Goodfellow and his colleagues in 2014. GANs consist of two neural networks – the generator and the discriminator – engaged in a continuous, adversarial training process. The generator creates synthetic data, and the discriminator evaluates it against real data. This dynamic interplay refines the generator's ability to produce increasingly realistic content.
The Why: Significance of Generative AI
Creative Content Generation: One of the primary applications of Generative AI is in the creation of content. From images and videos to text and music, GANs have the capacity to generate content that is often indistinguishable from that created by humans. This has transformative implications for industries such as entertainment, design, and marketing.
Data Augmentation and Synthesis: Generative AI excels in data augmentation, generating additional training data to enhance model performance. In scenarios where acquiring large datasets is challenging, GANs can create synthetic data, enabling more robust machine learning models.
Realistic Simulation and Training: GANs are instrumental in creating realistic simulations for training purposes. In fields like autonomous vehicles, healthcare, and aviation, Generative AI can simulate diverse scenarios, allowing models to learn and adapt to various conditions without real-world risks.
Anomaly Detection and Fraud Prevention: The ability of Generative AI to recognize patterns makes it invaluable for anomaly detection. In the financial sector, for example, GANs can identify irregularities in transactions, enabling timely intervention and fraud prevention.
Human-Like Conversational Agents: Natural Language Processing (NLP) powered by Generative AI has paved the way for human-like conversational agents. Chatbots and virtual assistants can engage in dynamic, context-aware conversations, significantly improving customer interactions and support services.
The How: Unveiling the Intricacies of Generative AI
- Architecture: Generator and Discriminator
At the core of GANs is a duelling pair of neural networks – the generator and the discriminator. The generator creates synthetic data, while the discriminator evaluates whether the data is real or generated. This adversarial relationship drives the continual improvement of the generator's ability to produce realistic outputs.
- Training Process: Adversarial Training
The training process begins with the generator creating synthetic data, which is then presented to the discriminator along with real data. The discriminator's role is to distinguish between the two types of data. As the generator improves, the discriminator must adapt, creating a cycle of continuous improvement for both networks.
- Loss Function: Striving for Equilibrium
The success of GANs relies on achieving equilibrium between the generator and the discriminator. The loss function, representing the error in the generator's output, guides the training process. When equilibrium is reached, the generator produces content that is so realistic that the discriminator struggles to differentiate between real and generated data.
- Applications: Diverse and Impactful
Generative AI finds applications across various domains. In image generation, StyleGAN has proven effective in creating lifelike portraits. OpenAI's GPT models, based on Generative Pre-trained Transformers, have set new benchmarks in natural language understanding and generation.
- Challenges: Ethical Considerations and Bias
While Generative AI holds immense potential, it is not without challenges. Ethical considerations, such as the potential misuse of deepfakes for malicious purposes, and the presence of bias in generated content, are critical issues that demand attention.
TransformHub: Harnessing Generative AI for Digital Transformation
For a deeper understanding of how Generative AI can be harnessed for Digital Transformation Services, let's turn our attention to TransformHub, a BFSI-specific company at the forefront of innovation.
- Tailored Customer Solutions
TransformHub utilizes Generative AI to understand customer behavior and preferences. By analyzing vast datasets, Generative AI models can offer personalized solutions that cater to individual needs, enhancing customer satisfaction and loyalty.
- Automated Regulatory Compliance
In the highly regulated BFSI sector, staying compliant with evolving regulations is a perpetual challenge. TransformHub leverages Generative AI to automate compliance processes, ensuring that the company and its clients remain in adherence to the latest regulatory requirements.
- Risk Mitigation Strategies
Generative AI models are employed by TransformHub to develop robust risk mitigation strategies. By analyzing historical data and market trends, these models can predict potential risks and opportunities, allowing for better-informed decision-making and strategic planning.
- Efficient Data Management
TransformHub optimizes data management processes through the integration of Generative AI. From data extraction to categorization, these models automate routine tasks, allowing BFSI clients to focus on strategic decision-making rather than tedious manual processes.
Conclusion: Paving the Way Forward
Generative AI stands as a testament to the boundless capabilities of artificial intelligence. Its impact spans across industries, with transformative applications that redefine how we generate and interact with data. In the BFSI sector, where precision, security, and innovation are paramount, Generative AI, when harnessed judiciously, becomes a catalyst for digital transformation.
As we navigate the intricate workings of Generative AI – the what, the why, and the how – the journey is not just a technological evolution; it's a paradigm shift. And with innovative companies like TransformHub leading the charge, the integration of Generative AI into Digital Transformation Services propels us into an era where possibilities are limited only by our imagination. The future is not just bright; it's generatively intelligent.
Share this
You May Also Like
These Related Stories
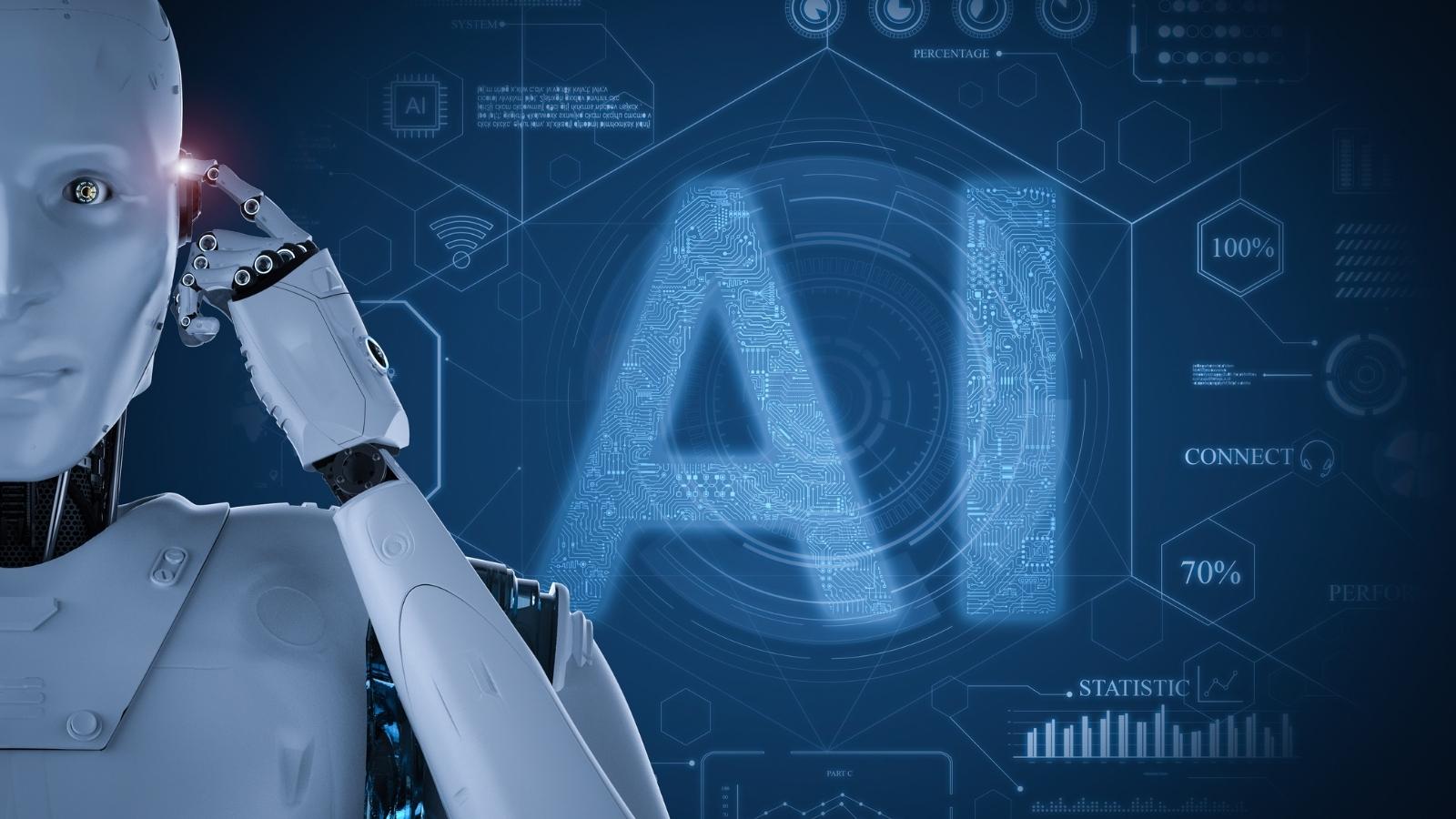
Why Robotic Process Automation in Insurance needs an AI upgrade
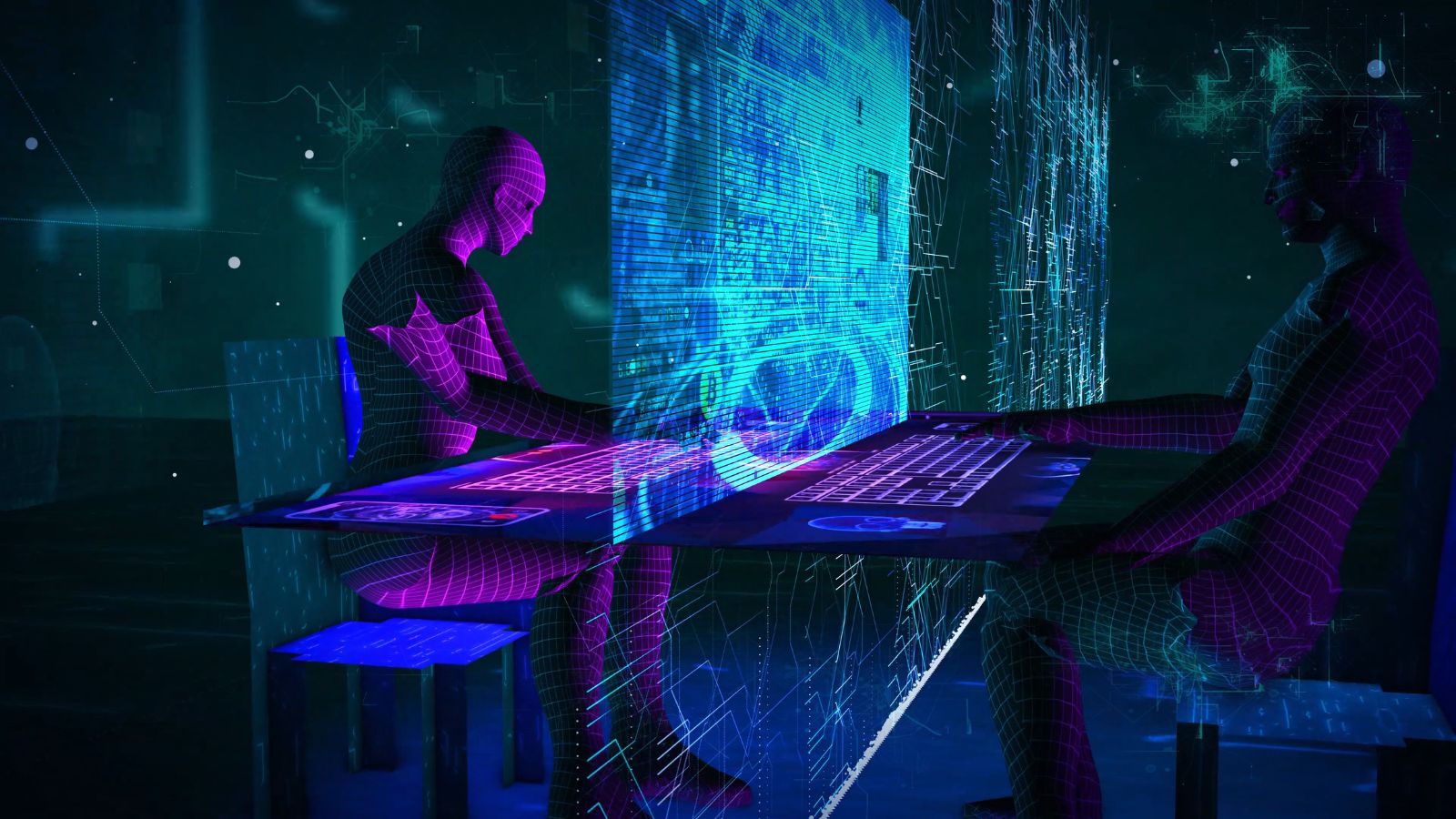
What is Data-Centric AI & How to Adopt This Approach
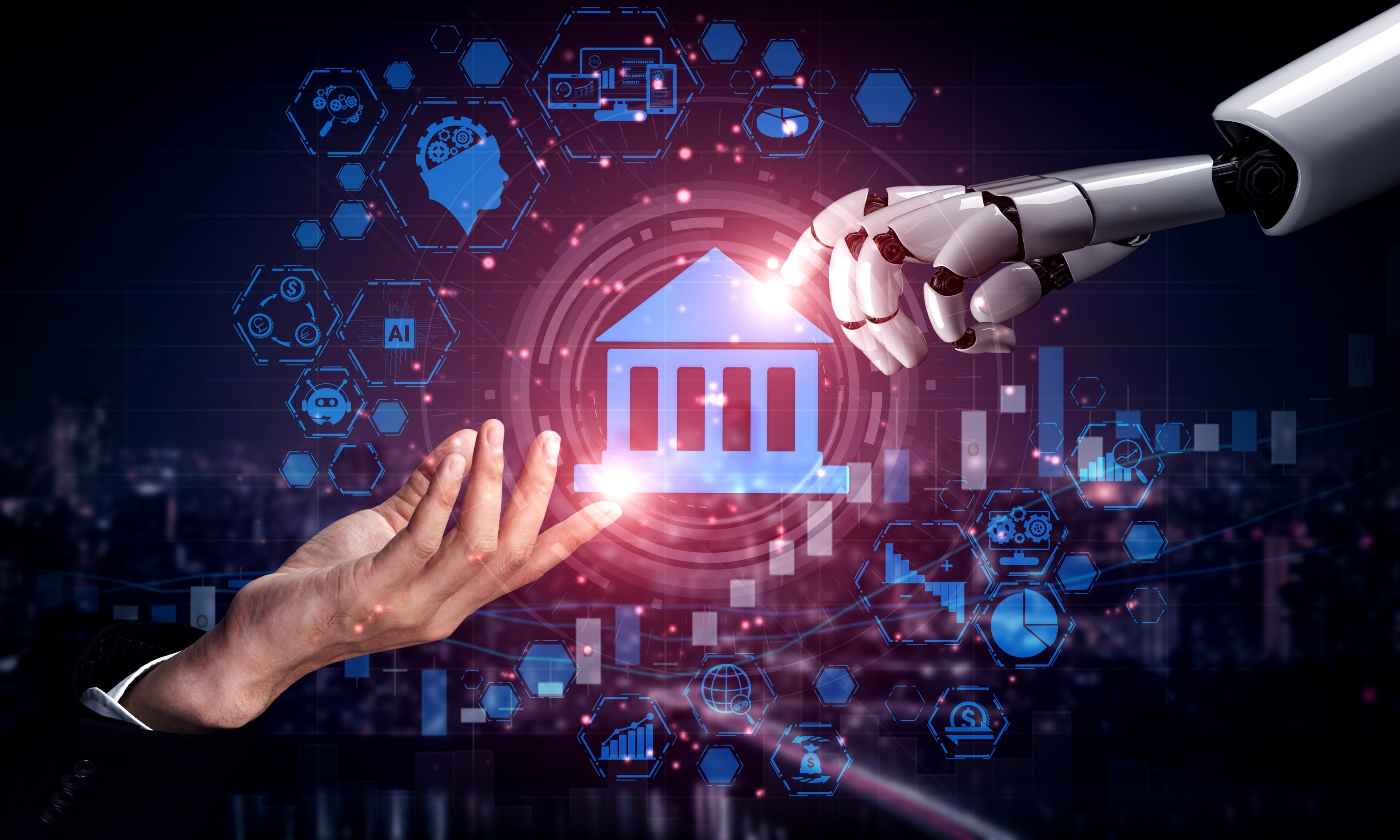